Introduction:
In a groundbreaking achievement, researchers at the Korea Advanced Institute of Science and Technology (KAIST) have successfully developed an artificial intelligence (AI) semiconductor capable of processing large language model (LLM) data at ultra-high speeds while significantly reducing power consumption. The achievement, announced by the Ministry of Science and ICT, introduces a novel complementary-transformer AI chip, representing a significant leap in neuromorphic computing technology.
Follow us on LinkedIn for everything around Semiconductors & AI
The Complementary-Transformer AI Chip:
Led by Professor Yoo Hoi-jun at KAIST’s processing-in-memory research center, the research team utilized Samsung Electronics’ 28-nanometer manufacturing process to create the world’s first complementary-transformer AI chip.
This neuromorphic computing system mimics the structure and function of the human brain, thereby paving the way for more efficient and powerful AI processing.
Read More:Deepsouth Awakens: The Human Brain-Scale Supercomputer Coming in 2024 – techovedas
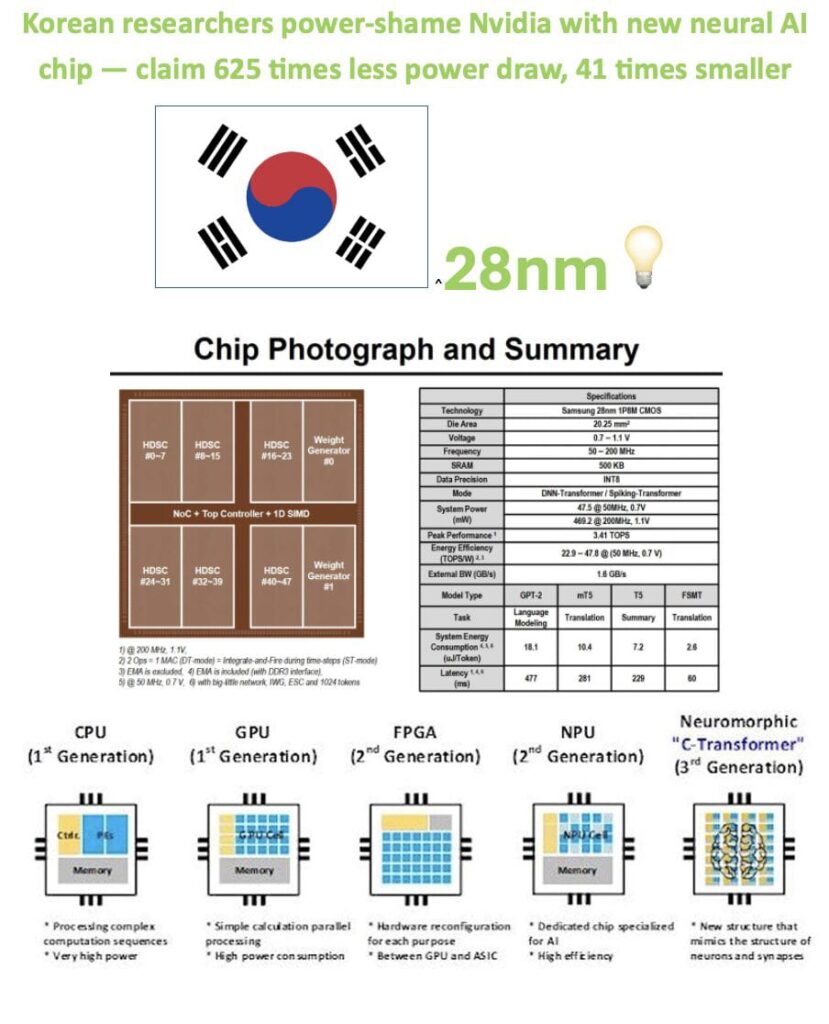
credits – Marco Mezger, Linkedin
Key Features:
Structure Inspired by Human Brain:
- The complementary-transformer AI chip is designed to emulate the intricate structure of the human brain, enabling it to process information in a manner similar to neurons.
Deep Learning Model Implementation:
- The research team successfully implemented a deep learning model commonly used in visual data processing, such as providing insights into how neurons process information.
Context and Meaning Learning:
- The technology employed allows the chip to learn context and meaning by tracking relationships within data, such as words in a sentence. This is a crucial feature for generative AI services like ChatGPT.
Demonstration and Performance:
The research team showcased the functionality of the complementary-transformer AI chip at the ICT ministry’s headquarters in Sejong.
Kim Sang-yeob, a member of the team, demonstrated various tasks such as sentence summarization, translation, and question-and-answer tasks using OpenAI’s LLM, GPT-2.
The chip, integrated into a laptop, exhibited remarkable performance enhancements, such as completing tasks at least three times faster and, in some cases, up to nine times faster than running GPT-2 on an internet-connected laptop.
Read More: US Urges Japan and Netherlands to Tighten Restrictions on China’s Semiconductor Access – techovedas
Power Efficiency and Compact Design:
One of the most remarkable aspects of this achievement is the chip’s ability to operate on significantly lower power.
While traditional implementations of large language models require substantial graphic processing units (GPUs) and 250 watts of power moreover, the KAIST team managed to implement the language model using a compact AI chip measuring just 4.5 millimeters by 4.5 millimeters.
This is a remarkable feat, as the semiconductor reportedly uses only 1/625 of the power and is only 1/41 the size of Nvidia’s GPU for the same tasks.
Significance for On-Device AI:
Professor Yoo Hoi-jun predicts that this technology could emerge as a core component for on-device AI, such as enabling AI functions to be executed within a device without relying on an internet connection.
On-device AI offers faster operating speeds and lower power consumption compared to cloud-based AI services, which depend on network connectivity.
Potential Impact on the AI Semiconductor Market:
The research team’s breakthrough could have a profound impact on the AI semiconductor market.
With the increasing demand for generative AI services and the growing need for on-device AI, innovative solutions such as the complementary-transformer AI chip could become a key player in the industry.
Conclusion:
KAIST’s successful development of the complementary-transformer AI chip marks a significant milestone in the field of AI semiconductors.
Additionally,the breakthrough not only demonstrates the capability of neuromorphic computing but also opens up new possibilities for on-device AI with enhanced speed and reduced power consumption.
As the research team continues to focus on addressing market needs, the technology could play a pivotal role in shaping the future of AI processing.