Introduction:
An AI accelerator, also known as a neural processing unit (NPU) or AI chip, is a specialized hardware component designed to perform the complex computations required for artificial intelligence tasks efficiently. Traditional CPUs (Central Processing Units) are not optimized for the parallel processing demands of AI algorithms, so AI accelerators are developed to handle these tasks more effectively. They can dramatically speed up AI workloads such as machine learning, deep learning, and neural network inference, making them crucial in many modern AI applications, from image recognition to natural language processing. These AI accelerator market consists of various devices, including smartphones, data centers, and autonomous vehicles.
The AI accelerator market is experiencing unprecedented growth, fueled by the escalating demand for high-performance hardware solutions to power artificial intelligence applications. In this blog post, we’ll delve into six companies that are spearheading innovation in this dynamic industry, highlighting their key contributions, strategic approaches, and unique offerings.
Follow us on LinkedIn for everything around Semiconductors & AI
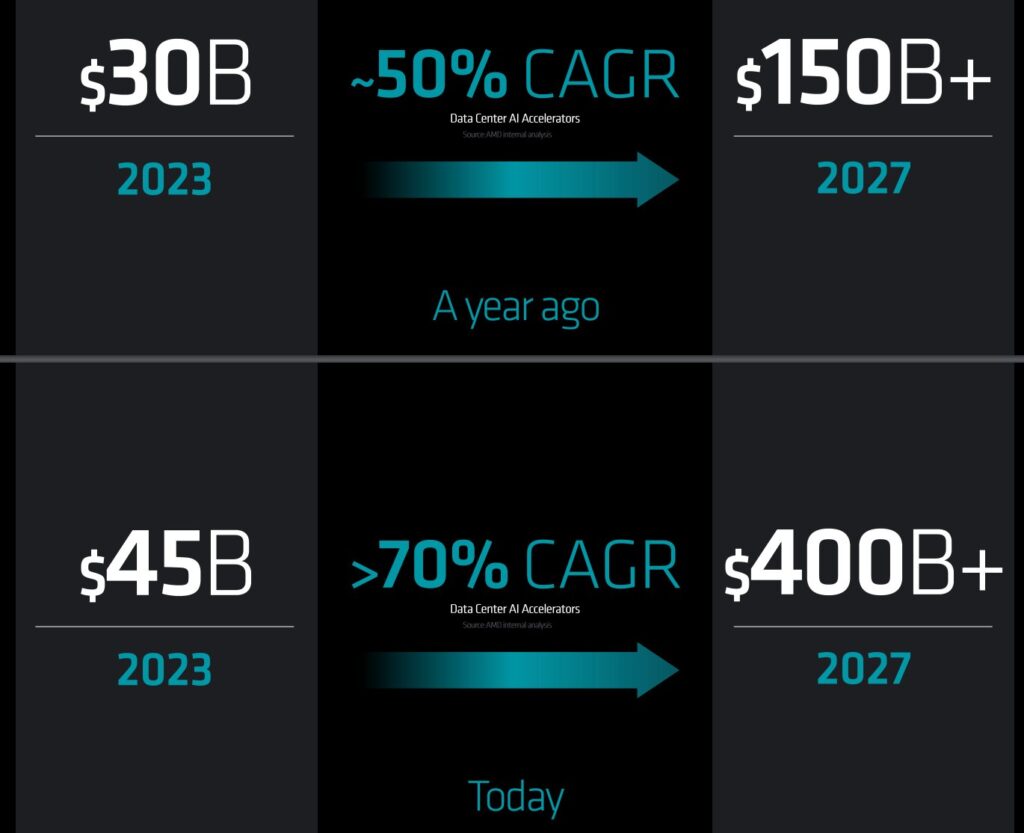
1. Nvidia & AMD:
Nvidia and AMD stand as two giants in the AI accelerator space, each bringing its own strengths to the table. While Nvidia has long dominated with its powerful GPUs, AMD is gaining ground with its Instinct MI300, slated for production by the end of 2023. Nvidia’s Hopper H100 boasts impressive performance and efficiency, but AMD’s MI300 promises to challenge its dominance with its versatile capabilities across a range of AI workloads.
Nvidia remains the leader in AI training, but AMD is a strong contender with its competitive pricing and open-source approach. The market is evolving, and the choice between the two depends on specific needs, budget, and software preferences.
Nvidia:
- Strengths:
- Market leader: Nvidia dominates the AI training chip market with its CUDA ecosystem and powerful GPUs like the A100 and H100.
- Mature software: CUDA is widely adopted and well-integrated with popular AI frameworks like TensorFlow and PyTorch.
- Strong ecosystem: Nvidia partners with cloud providers and hardware vendors, offering robust support and integration.
- Weaknesses:
- High cost: Nvidia’s solutions are generally more expensive than AMD’s.
- Closed ecosystem: Reliance on CUDA can limit developer flexibility and adoption.
- Focus on training: Nvidia primarily targets the training market, offering limited options for inference workloads.
AMD:
- Strengths:
- Competitive pricing: AMD’s AI accelerators, like the Instinct MI300, are often more affordable than Nvidia’s offerings.
- Open-source approach: AMD promotes ROCm, an open-source alternative to CUDA, fostering broader compatibility and innovation.
- Growing presence in inference: AMD is expanding its reach in the inference market with dedicated solutions like the Alveo V70.
- Weaknesses:
- Market share gap: AMD still holds a smaller market share compared to Nvidia.
- Software maturity: ROCm is less mature than CUDA and requires more developer effort.
- Limited ecosystem: AMD’s partner network is currently smaller than Nvidia’s.
Current Trends:
- AMD is making significant strides, recently showcasing impressive performance gains in benchmarks.
- Cloud providers are starting to adopt AMD’s solutions, offering customers more choice and potentially driving competition.
- The focus is shifting towards inference, where AMD could potentially gain ground due to its competitive pricing and open-source approach.
2. Microsoft vs. Google:
Microsoft and Google are key players in the AI accelerator market, but their approaches differ significantly. Microsoft’s Maia 100, developed in collaboration with OpenAI, targets large language models and cloud-based AI applications, catering to enterprise needs. On the other hand, Google’s Tensor Processing Units (TPUs) have been at the forefront of AI hardware development since 2015, offering accelerated performance for a wide range of workloads, both internally and through Google Cloud.
Microsoft:
- Focus: Cloud-based AI inference with FPGAs (Field-Programmable Gate Arrays) and custom ASICs (Application-Specific Integrated Circuits).
- Strengths:
- Azure cloud integration: Optimized AI acceleration within the Azure platform for seamless deployment and scalability.
- Customizable hardware: FPGAs and ASICs offer flexibility for specific workloads and performance needs.
- Partnerships: Collaborates with Intel and others to expand hardware options and solutions.
- Weaknesses:
- Limited focus on training: Primarily concentrates on inference, leaving a gap in the training accelerator space.
- Less open-source: Hardware and software offerings might be less open compared to Google’s approach.
- Smaller market share: Currently holds a smaller market share compared to Google in AI accelerators.
Google:
- Focus: Open-source hardware and software for both training and inference, using TPUs (Tensor Processing Units).
- Strengths:
- TensorFlow integration: Tightly coupled with TensorFlow framework, simplifying development and deployment.
- Open-source TPU design: Encourages community innovation and fosters wider adoption.
- Market leader: Holds a larger market share in AI accelerators overall, driven by TPU adoption.
- Weaknesses:
- Limited cloud integration: TPUs primarily used in Google Cloud, hindering broader utilization on other platforms.
- Hardware availability: Limited access to physical TPUs for smaller players or individual developers.
- Software complexity: Utilizing TPUs effectively might require deeper technical expertise.
Current Trends:
- Both companies are actively improving their offerings. Microsoft’s latest Project Brainwave FPGAs show promising performance gains.
- Open-source hardware gains traction, potentially challenging Google’s market dominance.
- Cloud platform integration remains crucial for widespread adoption and ease of use.
Read More: What are 5 Techniques in Advanced Packaging?
Amazon vs. Tesla:
Amazon and Tesla represent two distinct approaches to AI acceleration, with a focus on cloud-based applications and autonomous driving, respectively. Amazon’s AWS Trainium and Inferentia accelerators offer cost-effective and scalable solutions for cloud-based AI workloads, catering to the diverse needs of AWS customers. In contrast, Tesla’s Dojo is designed to revolutionize neural network training for self-driving cars, leveraging Tesla’s expertise in AI and automotive technology.
While both Amazon and Tesla are major players in the tech industry, their approaches to AI accelerators differ significantly. Here’s a breakdown:
Amazon:
- Focus: Cloud-based AI inference with custom-designed NPUs (Neural Processing Units) like Trainium and Inferentia.
- Strengths:
- Tight integration with AWS: Seamless deployment and scaling within the AWS cloud platform.
- Focus on cost-performance: Competitive pricing for their NPU offerings.
- Growing ecosystem: Partnerships with various hardware and software vendors expand their reach.
- Weaknesses:
- Limited availability: Physical access to their NPUs might be restricted to select users.
- Less open-source: Primarily focused on proprietary hardware and software solutions.
- Newer entrant: Compared to Tesla, Amazon has less experience in the dedicated AI accelerator market.
Tesla:
- Focus: Developing their own AI hardware for internal use (e.g., self-driving cars) and limited external offerings. Their DOJO D1 chip focuses on training, while the Tesla Autopilot HW4 targets inference.
- Strengths:
- Cutting-edge technology: Known for pushing the boundaries of hardware performance.
- Vertical integration: Design and manufacture both hardware and software, enabling tight optimization.
- Real-world validation: Extensive testing and use of their AI hardware in Tesla vehicles.
- Weaknesses:
- Limited external availability: DOJO D1 is currently not commercially available, and HW4 is exclusive to Tesla vehicles.
- Closed ecosystem: Primarily focused on proprietary solutions, limiting broader adoption.
- Less focus on cloud integration: Their offerings cater more towards on-premise deployments.
Read More: 6 Leading Companies in Advanced Packaging of Integrated Circuits – techovedas
Conclusion:
Intense competition and rapid innovation define the AI accelerator market, as companies vie for leadership. Nvidia and AMD push performance and efficiency boundaries, while Microsoft and Google leverage partnerships and cloud-based solutions to meet evolving customer needs. Meanwhile, Amazon and Tesla are pursuing distinct approaches, with a focus on cloud-based applications and autonomous driving, respectively. As demand for AI accelerators continues to soar, these companies are poised to play a pivotal role in shaping the next chapter of AI innovation.