Introduction
Nvidia’s H100 AI GPUs are taking the tech world by storm, but their reign comes at the price of a hefty energy bill. According to Stocklytics.com, these power-hungry processors are projected to consume a staggering 13,797 GWh in 2024, exceeding the annual energy consumption of nations like Georgia and Costa Rica.
These findings bring up concerns about the environmental impact and sustainability of this AI advancement.
Follow us on Linkedin for everything around Semiconductors & AI
Understanding NVIDIA’s Energy Appetite
The heightened power density of high-end GPUs presents substantial new challenges for data center planning, as it necessitates a fourfold increase in power supply capacity to adequately support modern AI data centers.
This shift in power demands significantly alters the landscape of data center infrastructure, requiring proactive measures to accommodate the escalating power requirements of GPU-driven computational workloads.
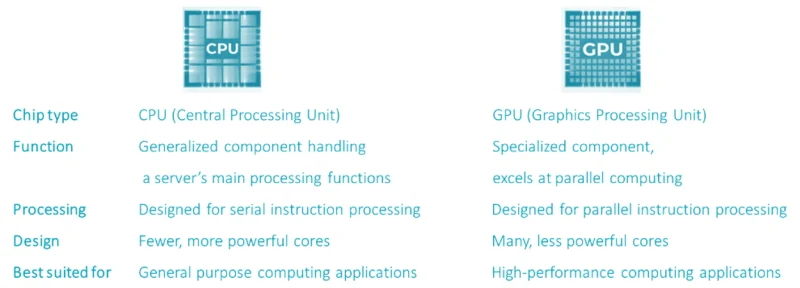
AI, which often requires running computations on gigabytes of data, needs enormous computing power compared with ordinary workloads. And, Nvidia’s cutting-edge H100 AI GPUs are leading the way with energy consumption of over 13,000 GWh this year. Each H100 GPU, running at 61% annual utilization, consumes roughly 3,740 kilowatt-hours (kWh) of electricity annually. This is equivalent to the average American household. While this figure might seem alarming, GPU efficiency may improve in the near future, offering a potential path towards more sustainable computing.
Read more Hardest Problem for Semiconductor & AI Industry: Energy Efficient Computing – techovedas
But why do GPUs consume so much power?
Data centre GPUs consume a substantial amount of power primarily due to their high computational requirements and the complex algorithms they handle.These GPUs optimize parallel processing tasks like machine learning and data analytics, involving simultaneous processing of vast amounts of data.
While parallel processing speeds up data processing, one demerit is that, at a time most parts of a chip are active. This is because the chip runs on a clock signal and the clock runs all the time. In applications requiring continuous operation, such as data centers, employing techniques like clock gating and power gating doesn’t yield significant benefits.
This constant computation, coupled with the execution of complex algorithms, demands significant computational power, thereby increasing energy consumption.
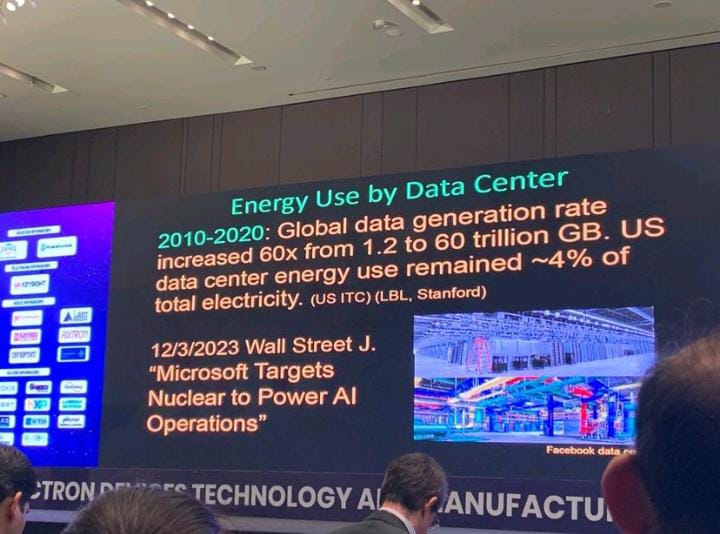
Still from IEEE EDTM’24 held at Bengaluru this year
The large-scale deployment of GPUs in data centers, where racks and clusters utilize hundreds or thousands of GPUs, further amplifies their collective power consumption. This combination of factors underscores the considerable energy consumption associated with data centre GPUs.
Read more AI goes Analog: How Analog AI chips are more energy efficient – techovedas
Energy challenge of powering AI chips
The AI revolution will require nothing short of a complete re-engineering of the data centre infrastructure from the inside out.
As Nvidia’s aspirations grow higher, concerns are emerging regarding the impact of the escalating energy requirements linked to its cutting-edge chip technologies.
According to Paul Charnock, Microsoft’s Principal Electrical Engineer of Datacenter Technical Governance and Strategy, the installation of millions of Nvidia H100 GPUs will consume more energy than all households in Phoenix, Arizona by the end of 2024.
Thus, successfully navigating these challenges and fostering innovation will shape the future landscape of AI computing and beyond.
Read more Why AI Needs a New Chip Architecture – techovedas
Nvidia ventures into a $30 billion tailored chip market
Nvidia, a leading player in AI chip design, is broadening its scope by venturing into custom chip development for cloud computing and AI applications. The firm is now looking to tap into the growing custom chip sector, projected to reach $10 billion this year and double by 2025. The broader custom chip market hit around $30 billion in 2023, accounting for 5% of chip sales annually.
Based in Santa Clara, California, Nvidia targets the changing needs of tech giants such as OpenAI, Microsoft, Alphabet, and Meta Platforms. The company is establishing a division focused on developing custom chips, including powerful artificial intelligence (AI) processors, for cloud computing firms and other enterprises.
Currently holding 80% of the high-end AI chip market share, the position has driven Nvidia’s stock market value up by 40% so far this year to $1.7 trillion after a more than threefold increase in 2023.
Promising solutions to this power consumption
Amazon’s recent unveiling of the Arm-based Graviton4 and Trainium2 chips holds promise for efficiency gains.
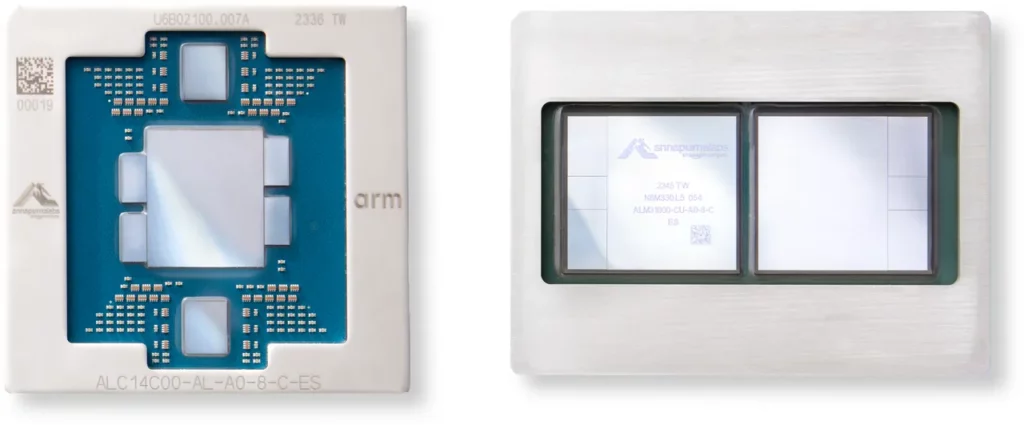
The Graviton 4 chip, left, is a general-purpose microprocessor chip being used by SAP and others for large workloads, while Trainium 2 is a special-purpose accelerator chip for very large neural network programs such as generative AI.
The chips are “designed to deliver up to four times faster training performance and three times more memory capacity” than their predecessor, “while improving energy efficiency (performance/watt) up to two times,” said Amazon.
Additionally neuromorphic computing is being researched aggressively as an alternative to synchronous parallel computing architectures. Neuromorphic computing is an asynchronous computing paradigm which runs on event based ‘spikes’ rather than a clock signal. This means only those parts of the chip are active which are currently processing an event. This drastically lowers the power consumption.
Read more Korean researchers power share NVIDIA with their new AI Chip – techovedas
Conclusion
As the tech world grapples with the energy challenges posed by Nvidia’s H100 AI GPUs, which are projected to consume a staggering 13,797 GWh in 2024, equivalent to the annual energy consumption of entire nation, the need for sustainable solutions becomes paramount. Innovations such as Amazon’s Graviton4 and Trainium2 chips are a ray of hope. Moreover, the exploration of neuromorphic computing, which drastically lowers power consumption through asynchronous processing, presents a promising alternative. By embracing these advancements, the tech industry can pave the way for a more sustainable method to process AI.